Last updated: October 15, 2022
Article
Mapping Arctic Alaska's Coastal Vegetation
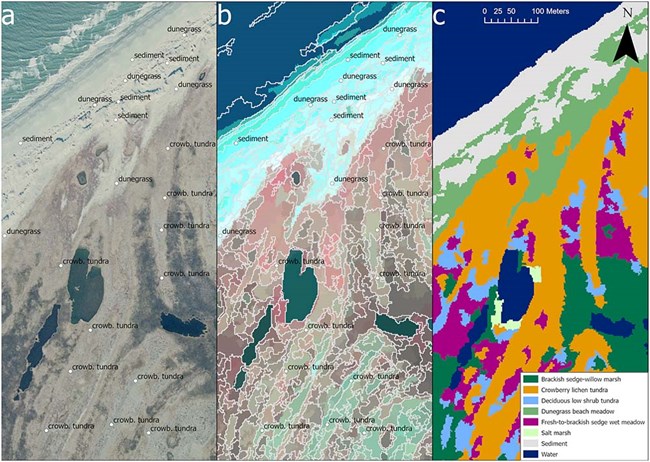
Imagery: Manley, WF, Sanzone, DM, Lestak, LR, and Parrish, EG 2007. Index Layers for High-Resolution Orthorectified Imagery from 2003 for the Coastal Areas of Bering Land Bridge NPres. https://irma.nps.gov/DataStore/Reference/Profile/1044493
Parks on the northwest coast of Alaska are remote and rugged. They also change due to sediment deposition or erosion, currents, storms, sea ice dynamics, and more. The Bering and Chukchi seas are also experiencing an increase in shipping traffic as the sea-ice-free season gets longer. With the potential for an oil spill, how would we know what coastal resources were damaged? We need a baseline. Mapping the coastal vegetation provides a baseline for any potential changes that may occur in the future.
In this study, researchers synthesized vegetation and geospatial data to create a new high-resolution coastal vegetation map for the coasts of Bering Land Bridge National Preserve and Cape Krusenstern National Monument. Using an approach that segments satellite imagery along natural boundaries, this paper offers an accurate and efficient way to classify vegetation, as well as a map that can be used to respond to oil spills and monitor coastal change.
A high-resolution map of coastal vegetation for two Arctic Alaskan parklands: An object-oriented approach with point training data
Abstract
Bering Land Bridge National Preserve and Cape Krusenstern National Monument in northwest Alaska have approximately 1,600 km of predominantly soft-sediment coastlines along the Chukchi Sea, a shallow bay of the Arctic Ocean. Over the past decade, marine vessel traffic through the Bering Strait has grown exponentially to take advantage of new ice-free summer shipping routes, increasing the risk of oil spills in these fragile ecosystems. We present a high-resolution coastal vegetation map to serve as a baseline for potential spill response, restoration, and change detection. We segmented 663 km2 of high-resolution multispectral satellite images by the mean-shift method and collected 40 spectral, topographic and spatial variables per segment. The segments were classified using photo-interpreted points as training data, and verified with field based plots. Digitizing points, rather than polygons, and intersecting them with the segmentation allows for rapid collection of training data. We classified the map segments using Random Forest because of its high accuracy, computational speed, and ability to incorporate non-normal, high-dimensional data. We found creating separate classification models by each satellite scene gave highly similar results to models combining the entire study area, and that reducing the number of variables had little impact on accuracy. A unified, study area-wide Random Forest model for both parklands produced the highest accuracy of various models attempted. We mapped 18 distinct classes, with an out-of-bag error of 11.6%, resulting in an improvement to the past per-pixel classification of this coast, and in higher spatial and vegetation classification resolution. The resulting map demonstrates the utility of our point-based method and provides baseline data for incident preparedness and change detection. Elevation is highly correlated with the ordination of the vegetation types, and was the most important variable in all tested classification models. The vegetation classification brings together the largest amount of vegetation data for the Chukchi Sea coast yet documented.
Hampton-Miller, C. J., P. N. Neitlich, and D. K. Swanson. 2022. A high-resolution map of coastal vegetation for two Arctic Alaskan parklands: An object-oriented approach with point training data. PLOS ONE 17(8): e0273893.