Last updated: April 1, 2024
Article
Sea otter distribution modeling
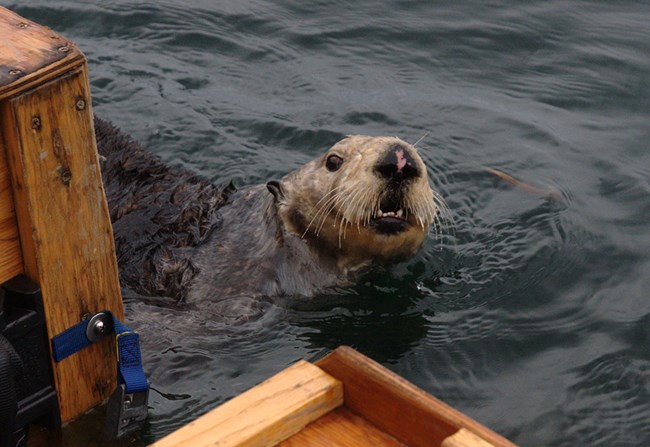
Predictive modeling is a common tool to quantify animal distributions and their habitat associations, but can be challenging in data-limited ecosystems. This study is a step forward in exploring habitat features that may be important for estimating sea otter carrying capacity in southcentral Alaska.
A component necessary for carrying capacity estimates is prey availability data, which influences equilibrium densities of sea otters. Despite large efforts to model carrying capacity in other regions, many studies, including this one, have not incorporated sea otter prey information directly, which is recommended for future modeling efforts. This study found that the most influential habitat attributes predicting sea otter occurrence in Kachemak Bay were bathymetry, shell litter, and filamentous algae, followed by understory kelp.
Identifying habitat requirements is important for species management and conservation. As this study has shown, it is promising that modeling species distributions and habitat associations is possible in data-limited ecosystems. Much is still unknown about our oceans and the ability to answer questions with open-access data can help fill key information gaps. Beyond sea otters, this framework can be beneficial for species distribution modeling of other mobile marine megafauna. Specifically, understanding the habitat requirements of protected species and predicting distributions can mitigate future conflicts of resource competition or disturbance. The minimal data requirements of animal presence and dispersed habitat data over an area of interest can lead to significant gains in knowledge for management and conservation by using MaxEnt for species distribution modeling in data-limited ecosystems.
Species distribution modeling of northern sea otters (Enhydra lutris kenyoni) in a data-limited ecosystem
Abstract
Species distribution models (SDMs) are used to map and predict the geographic distributions of animals based on environmental covariates. Typically, SDMs require high-resolution habitat data and time series information on animal locations. For data-limited regions, defined as having scarce habitat or animal survey data, modeling is more challenging, often failing to incorporate important environmental attributes. For example, for sea otters (Enhydra lutris), a federally protected keystone species with variable population trends across the species' range, predictive modeling of distributions has been successfully conducted in areas with robust sea otter population and habitat data. We used open-access data and employed a presence-only model, maximum entropy (MaxEnt), to investigate subtidal habitat associations (substrate and algal cover, bathymetry, and rugosity) of northern sea otters (E. lutris kenyoni) for a data-limited ecosystem, represented by Kachemak Bay, Alaska. Habitat association results corroborated previous findings regarding the importance of bathymetry and understory kelp as predictors of sea otter presence. Novel associations were detected as filamentous algae and shell litter were positively and negatively associated with northern sea otter presence, respectively, advancing existing knowledge of sea otter benthic habitat associations useful for predicting habitat suitability. This study provides a quantitative framework for conducting species distribution modeling with limited temporal and spatial animal distribution and abundance data. Utilizing drop camera information, our novel approach allowed for a better understanding of habitat requirements for a stable northern sea otter population, including bathymetry, understory kelp, and filamentous algae as positive predictors of sea otter presence in Kachemak Bay, Alaska.
Hasan, E. L., K. B. Gorman, H. A. Coletti, and B. Konar. 2024. Species distribution modeling of northern sea otters (Enhydra lutris kenyoni) in a data-limited ecosystem. Ecology and Evolution 14(3): e11118.